How to Use Machine Learning Without Being a Data Scientist

Modern companies understand the value of data. Data allows factories and plants to run their processes more efficiently to increase capacity and quality.
It also allows them to organize their maintenance work effectively to reduce downtimes and get the most value out of their assets. These improvements come together to reduce the company’s costs and increase its profit margins.
At least, that’s the idea. Many companies are collecting more data than ever before from their manufacturing processes but they are struggling to convert the data into valuable, actionable information.
Unfortunately, raw data has to be analyzed and processed to yield the insights that will actually help a company improve its processes.
Until recently, advanced data analytics was a highly specialized skill that was not available to many manufacturing companies.
In this article, we will show you how recent advancements in machine learning are making it easier to apply machine learning to manufacturing data to extract insights from data and use those insights to make informed decisions on maintenance and quality monitoring.
After showing you how machine learning is already being applied in manufacturing, we will explain how you can use software solutions to build up the data collection and analysis capabilities on your factory floor today.
In this part, we will talk about machine learning-powered solutions and solutions that involve traditional data collection and monitoring.
Before we look at how machine learning can be applied to the factory floor, let’s talk about the problems that modern factories and plants face.
Problem
Most machines running on the shop floor are designed to report failures, not to predict them. Control systems are used to collect and process the data from the machines in real-time.
HMIs and SCADA systems are designed to show what is happening in the manufacturing process right now or in the past. They cannot tell you what will happen in the future.
That’s a shame because every failure that occurs can lead to expensive downtimes that halt production.
Some manufacturers have worked around this problem by implementing a preventative maintenance system. With preventative maintenance, you replace parts of a machine long before they fail to avoid production outages.
This strategy helps to avoid production outages but it is wasteful and it increases costs. It is very expensive to replace parts that are working and may continue to work for a long time with new parts.
Machine learning enables manufacturers to move from a preventative maintenance system to a predictive maintenance system.
With predictive maintenance, you use an algorithm based on historical data to determine if a part will fail soon and replace it as late as possible.
This strategy avoids production failures by replacing parts when required and reduced costs by only replacing parts that actually need to be replaced.

Unfortunately, most machines and process equipment are not designed to handle machine learning algorithms.
Although this equipment generates all of the data required to feed an algorithm, they do not have the processing capabilities to run these algorithms.
Even if they did, the engineering staff at these companies do not have the know-how to realize a complex machine-learning algorithm that accurately models the process anyway.
Data analysis and interpretation
Right now, many companies have started implementing a digitalization strategy where they use modern technologies like Single Pair Ethernet to collect data from the factory floor, IoT gateways to transmit the data, and on-premise or cloud-based databases to store the data.
As the pool of available data grows, the challenge becomes the interpretation of the data.
How can manufacturing companies analyze and interpret their data to improve their production processes and maintenance systems?

Solution
Although there are many different ways to analyze large data sets, machine learning is quickly emerging as the most efficient and cost-effective way to generate results.
In other industries, machine learning is revolutionizing the way data is analyzed and decisions are made. Companies have already seen that machine learning can create real value from collected data.
The manufacturing industry has been slow to deploy machine learning technology because it has always been difficult to do.
Until recently, using machine learning on your data involved hiring a team of data scientists to build a bespoke model, waiting for months while they trained the model, and eventually getting some results from the project.
The problem with this approach is not just that data scientists are specialist resources that are difficult to find.
Since data scientists are so rare, it has been hard for manufacturing companies to hire people with the right combination of data science skills and manufacturing knowledge.
What was required to deploy machine learning on the factory floor was an easy-to-use software that allows engineers to build, train, and deploy machine learning knowledge without data science skills.
Machine learning software
That is exactly what Weidmüller has delivered with their automated machine learning software Industrial AutoML.
It is a SaaS product that enables anyone to easily train machine learning algorithms on their data and deploy those algorithms either in the cloud or on the factory floor.
Training machine learning models in Industrial AutoML is an easy, three-step process.
You upload your data to Industrial AutoML and the tool visualizes your data in a time series graph.
In your visualized data, you use your domain knowledge to identify normal and abnormal data points.
Once your data has been identified as normal or abnormal, the tool creates a range of machine-learning data models. Once again, you can use your process knowledge to pick the best model based on performance and plausibility.

Finally, you can deploy the trained model in the cloud or on-premises to detect and predict anomalies. This trained model can help to improve processes and trigger the maintenance process to avoid downtime and reduce costs.
Also for machine builders the use of machine learning enables development of data-driven services, that helps the end-user.
This will reduce efforts in maintenance and support and improves quality and efficiency of the production process. It is a key differentiator for the machine builder compared to classical approaches.
Opportunities
When people hear about machine learning on the factory floor, they tend to think that this is a technology that is under development and will be available in the future. But the technology is here and ready to be used today.
Many innovative companies are already using machine learning on the factory floor to prevent downtime, improve the efficiency of processes, and reduce waste.
In this link, you can see one example of how Weidmüller is using AutoML at their Galvanic factory to monitor and predict maintenance requirements of a large number of fans and pumps using powerful machine learning algorithms.
To see how a machine builder explains the use of Industrial AutoML to create new data-driven services check this link.
What is needed to deploy machine learning?
Although machine learning technology is available to use today, manufacturing companies need to have a data infrastructure in place before they can leverage it.
For most manufacturers, there are three steps on the road to machine learning.
The first step is data collection. In this step, companies will upgrade their existing infrastructure to collect, process, store, and visualize the data that is being generated on the factory floor.
Tools like Weidmüller’s PROCON-WEB are built on modern web technologies and can be used to collect data from any type of PLC or controller and store it in an efficient database called a historian.
Once data is being collected, manufacturers can use software tools to monitor their data in real time. When seeking to improve processes and efficiencies, companies need to monitor more than production data.
Some visualization systems allow you to combine modular software solutions to also improve your energy and resource management.
Weidmüller is offering a modular Industrial IoT software kit that can be easily integrated into customer environments.
In addition to PROCON-WEB, you can install ResMa®, to record and monitor energy flows to find inefficiencies in your energy usage.
Once you are collecting and monitoring your data, you can use this data to train and implement machine learning algorithms that discover insights that you could not find for yourself and accurately predict the future based on your historical data.
By using PROCON-WEB, ResMa, and Industrial AutoML combined, those three steps can be done with little effort, since the software tool can be seamlessly combined.

Wrap-Up
Digitalization is enabling manufacturing companies to collect huge amounts of data from the factory floor.
The next challenge for manufacturers is to interpret this data and convert it into useful, actionable information. Machine learning is an effective and cost-effective way to do that.
Traditionally, it has been hard to deploy machine learning on the factory floor due to high barriers to entry.
It has been difficult for manufacturing companies to hire the teams or data scientists that are required to build, train, and maintain machine learning algorithms.
Modern tools like Industrial AutoML by Weidmüller have reduced that barrier to entry and made it easy for manufacturing companies to leverage machine learning on the factory floor.
These tools let any machine and process experts easily build, train, and deploy machine learning algorithms on-premises or in the cloud to monitor their production processes and predict when anomalies will occur.
By leveraging these modern tools and machine learning algorithms, manufacturers can improve their production and maintenance processes, leading to reduced costs and increased profit margins.
For machine builders offering data-driven service is a key differentiator in a highly competitive market.
To see in detail how you can deploy machine learning algorithms on the factory floor, check out the detailed AutoML tutorial from Weidmüller.
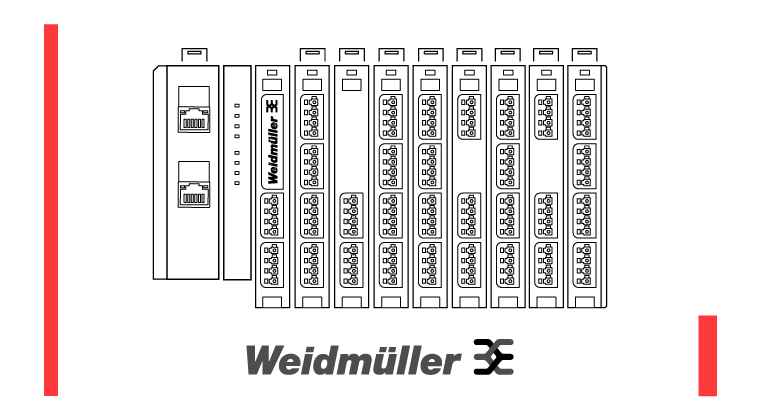
This course is designed to teach you everything you need to know to develop industrial IoT applications with Wedimüller’s u-mation platform.
Frequently asked questions
Learn from Industry Experts
With a 7-day trial, then €35/month